Expert Guide to Data Warehousing: What Are the Uses & Benefits of Data?
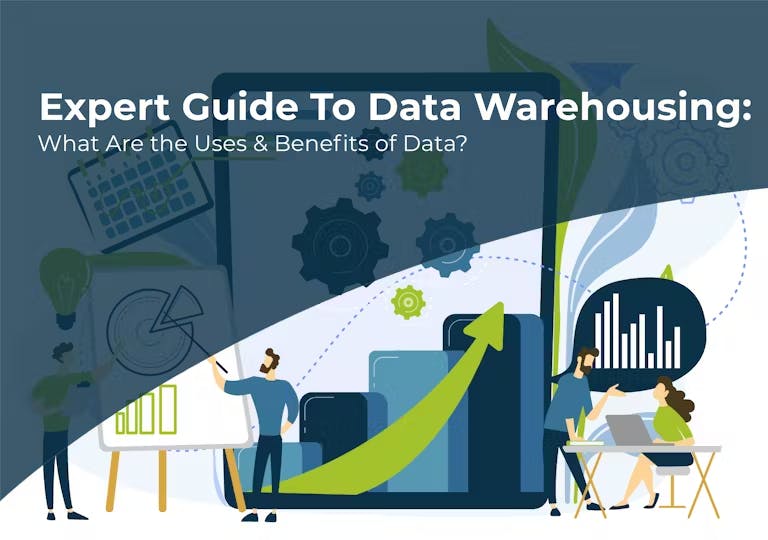
Business decisions rely on a variety of things, from market demands to company culture and mission statements. But the top business executives know that data-supported decisions are always going to take the lead. That’s why you see Tesla, Spotify, Nintendo, and other big brands using data analytics to empower their decision-making process.
Despite that, the chances are that you have data from multiple sources waiting to be analyzed and applied to your company. The International Data Corporation (IDC) estimated that 80% of data will be unstructured by 2025, and Forbes reported that 90% of unstructured data is never analyzed. This is up from 80% as recently as 2019, but when you consider the wide range of data sources found across any company, it’s not surprising that large amounts of unstructured data would pile up.
So, how can you use structured data warehousing for better business intelligence that puts you ahead of the competition? Let’s start with the basics, then move on to specific data analysis applications that can help unlock your potential.
What is a Data Warehouse?
Typically structured for organizational purposes, data warehousing (DWH) is defined by Astera as data stored digitally for company reports and searches. It’s most often for advanced reporting on business analytics or accessing data as needed. Data warehouses are meant for robust analysis, so they shouldn’t be confused with operational databases such as Microsoft SQL Server, MongoDB, and Apache Cassandra.
Most databases are for a specific purpose, like daily records of transaction systems, meaning they deal in real-time data exclusively. A data warehouse will store data from multiple sources and include historical data, which translates to more complex reporting for savvy business decisions. The journey of data, from source to warehouse, follows these steps:
- Data source: Think flat files, operation systems, etc. Exact data sources can vary by industry and how they do business. Data pipelines run from the source to staging.
- Staging area: Much like a physical warehouse, this area is for processing incoming data before sending it to be stored in the warehouse.
- Data warehouse: This is the centralized repository of information that’s been sorted and analyzed for easy navigation and reporting.
- Data marts: Divided into departments like sales or operations, data marts help with focused searches and reports for more precise analytics.
What Are the Benefits of Data Warehouses?
Top-notch data quality:
Your decisions are only as good as your data integrity, and Gartner research uncovered that poor data quality costs organizations $9.7 million each year. In America alone, IBM found that the total cost to businesses every year is $3.1 trillion in all. Data warehousing will protect your business from these steep costs. Rather than have your system down for long periods of time to clean up data, you can maintain it daily in an efficient process.
Increased productivity:
Instead of your team wasting precious time trying to navigate data scattered around your business, all in different formats, data warehousing makes it simple. All data is structured the same way in a warehouse. Your employees can get in, find what they need, and be on their way to data-driven decisions faster than ever before.
Accessibility and security:
Convenience paired with role permissions gives you the best of both worlds. Your staff can access the data warehouse easily, but only the people who need to edit the information (to remove duplicates and other tasks) can do so. Everyone else will have roles that let them see—but not alter—your stored data.
Scalability options:
With cloud data warehouses, you have essentially as much room as you need for data storage. You can expand capacity on demand for a better price than scaling up an on-premise data warehouse. There are pros and cons to both, of course. For example, if you need a specific tech stack, on-premise is the way to go. But having options is a benefit in itself.
Read: Achieve Faster Time To Market With DevOps & Cloud Computing Services
How Do Businesses Use Data Warehouses?
It’s important to understand that data warehouses have a wealth of applications across industries: healthcare, retail, hospitality, education—and plenty more. They all handle a lot of information and need to analyze it quickly and thoroughly. The common use cases listed below are the tip of the data iceberg, so bear that in mind if you choose data warehousing for your business. There’s always more opportunities to grow and adapt.
Performance Assessments:
Using metrics from the data warehouse, you can create reports or dashboards that provide updated and accurate performance results for your team. Whether you measure a successful day in orders completed, repairs made, or another KPI, you can have all the assessment details you need with data warehousing.
Marketing/Sales Campaigns:
When you have all your operation data in one place, your reports are timely and up-to-date, so you can meet your customers’ demands faster and more efficiently. Campaign performance can be measured in return on investment (ROI), customer acquisition costs, and more. Because this data is updated in real-time, the marketing team will understand your clientele’s current needs—and how to meet them.
Enterprise Data Warehouse (EDW):
A big company has to think about decision-making on a large scale, generally across hundreds or thousands of employees. That’s where enterprise data management delivers results. Some of the benefits include early risk assessment and cross-organizational data, so your business is communicating clearly and staying on track.
Machine Learning and AI Automation:
Around the world, only 1% of the datasphere is used for AI according to IBM. The potential for growth with AI technology is immense because it’s the next frontier for digital transformation. And the gateway for it? You guessed it: data warehousing. Forrester has called this a system of insight because the data stored in your warehouse can be integrated with machine learning and other AI tools for more accurate predictions. Since predictive tasks can ignite productivity in your workplace as it is, the perks are worth pursuing.
Analysis for Large Amounts of Data:
Where big data involves storing a large volume of information, it’s not as purpose-driven as data warehouses. Large data streaming makes sure unstructured data is analyzed before storage, so you don’t wind up with mountains of data that could be outdated and low quality. This data processing is incredibly fast, often done in real-time with a cluster of servers. Volume is the main factor here, with data sources typically being social media, purchase history, and other high-traffic data pipelines. The goal is to learn about service usage and activity, including website clicks as an example, for improved business intelligence and decisions.
Internet of Things (IoT) Data Integration:
Network-connected devices are called IoT devices, since they make their own physical network exchanging data between each other. Some examples include security devices, smartwatches, and more. Analyzing the vast amount of information they share and generate shows companies how to improve their processes for better results. By compiling historical and real-time analysis, you can predict trends based on past events and present influences. Internet of Things data integration even has the ability to detect anomalies.
Merging Legacy Data:
The name might sound prestigious, but legacy data technically means the information is kept in old formats or outdated storage systems. If you can’t easily access your information, you’re definitely not going to be able to process it. And generally speaking, the older the legacy software, the harder it would be to migrate that data to a newer one. But when you need historical data for reference and trend analysis, it’s vital to your operations. So, how do you take care of that? Extract, transform, and load (ETL) process lets you collect the data by changing these files into a newer format that your data warehouse can store and analyze.
Where to Begin with Data Warehousing
Data science and warehousing require adaptive technologies and expert personnel to get the job done. To make sure you have everything you need for your data transformation, talk with us today to go over specs and your project vision. We’re here for you.
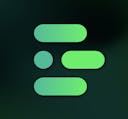